Blog
Artificial Intelligence Large Language Models (LLMs) and MGAs – Friends, Frenemies, or Foes?
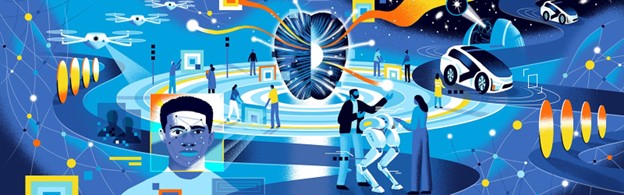
By 2025, it’s estimated that there will be up to 1 trillion connected devices. What do you see in the image other than the artificial intelligence lurking within the Internet of Things (IoT) just waiting to devour all the data. Oh wait that’s just Mission Impossible: Dead Reckoning and a different post, never mind. Source: Caltech Science Exchange, Artificial Intelligence.
Artificial intelligence (AI) has made it into the standard English vernacular, to be applied to everything and everyone – if watercooler talk has anything to say about it. We’ve blogged about it as well, in the setting of a potential copilot for underwriters. In my field, Andy Beck, a pathologist at Harvard, founded a company (PathAI) based on the finding that while the performance of physicians in diagnosing metastatic breast cancer in lymph nodes were 96% accurate and AI was 92%; the combination of both yielded 99.5% accuracy.
A pretty strong argument for thinking about AI copilots, I would say.
Here, we dial back the microscope and refocus to see more broadly. How much could AI-powered LLMs – like GPT, Claude, Llama, Gemini – potentially help the insurance industry and specifically MGAs? How can we move forward, and assimilate?
"... about 19% of American workers could see 50% or more of their tasks affected by LLMs."
A recent study by the kindly folks at Open AI and the University of Pennsylvania reported that about 19% of American workers could see 50% or more of their tasks affected by LLMs. They highlighted those tasks well aligned with LLM capabilities (e.g. writing, programming, data analysis) that could be integrated with software, would most greatly enhance operational efficiencies.
Well darn it, sign me up.
How can we integrate LLM + software into the insurance industry as a whole?
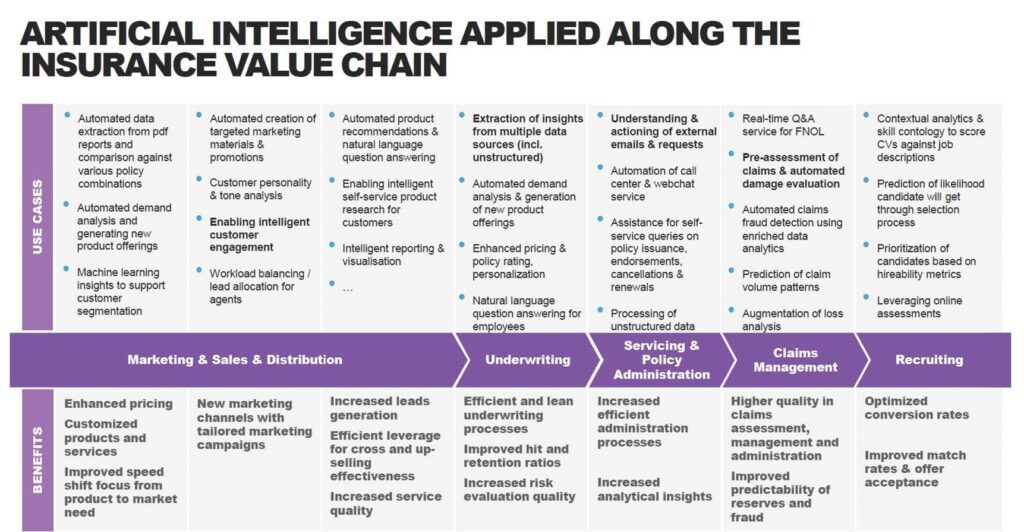
The many ways AI can be applied. Source: Accenture. Insurers: Start Boosting your “AIQ”. Last accessed 9/30/2024.
As you can see from Accenture’s graphic above, there’s more than what we have time to go into. But we’ll try to touch upon topics highly relevant to the industry, then focus down on how MGAs can strategically move forward with AI as a sidearm.
1. Underwriting
We’ve blogged about underwriting copilots before. But to reiterate and go a little bit deeper, AI-powered LLMs can significantly enhance the underwriting process by quickly analyzing vast amounts of structured and unstructured data, such as historical claims, financial reports, and market trends. This superhuman ability to synthesize complex information enables more precise risk assessment and pricing strategies. For example, platforms like Lemonade use AI-driven data analysis to customize premiums and provide instant quotes, a capability that LLMs can amplify to the nth power. Thanks NVIDIA!
The AI’s superpower is that it can predict subtle or previously unknown risk patterns that may not be immediately obvious to human underwriters. This could result in new rating algorithms that cause actuaries to low-key panic. But to start with, AI-powered LLMs should be used to reduce the time required to process more standard cases (read: higher numbers to train AI on), allowing underwriters to focus on complex, high-value accounts that require gray-area judgements.
2. Claims Management
Claims management is an area where LLMs can have a transformative effect. By automating initial claim intake, verifying data, and processing straightforward claims autonomously, LLMs can dramatically reduce response times and administrative costs.
Of course, it’s not always summer when the living is easy. For instance, State Farm experimented with using AI to streamline claims processing, but unfortunately got into some trouble when the algorithm was found to be discriminatory against black homeowners – which is a type of LLM bias we red-flagged previously.
But as long we understand how to appropriately train, LLMs can certainly handle more complex claims, co-piloting with claim experts by highlighting key data points, suggesting precedents, and even recommending settlement amounts based on historical data patterns. This hybrid (somewhat cybernetic) approach enables faster and more consistent outcomes while maintaining human oversight for nuance.
3. Operational Efficiency and Customer Service
LLMs can now reliably automate repetitive tasks such as data entry, policy renewals, and customer inquiries, enabling operations teams to reallocate resources – in keeping with the future of work. Chatbots and virtual agents like Salesforce’s Einstein Bots, are already being used to handle routine customer interactions, freeing up human agents to focus on more complex, emotionally sensitive issues.
Also, by integrating LLMs into backend systems, one could facilitate communication between departments, reducing information silos and improving the overall efficiency of internal processes.
But what can LLM’s do for Managing General Agents (MGAs)?
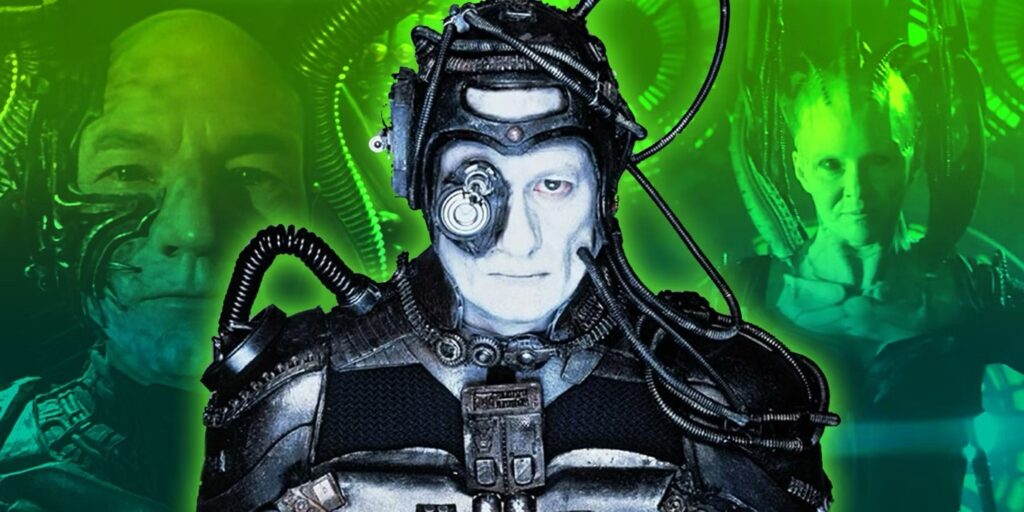
A possible future of highly, highly efficient work, if Elon and Mark have their way. Source: CBR.com, The History of the Borg – Star Trek’s Unstoppable Villains.
To gain a competitive edge, MGAs must take note of how the future of insurance will meld with artificial intelligence. MGAs should strategically incorporate LLMs into their business models by focusing on at least the following areas:
1. Enhancing Risk Assessment Capabilities
Invest into AI tools that enable deeper, more granular risk assessments. This would include leveraging LLMs to process alternative data sources (e.g., social media, economic reports) and to identify emerging risk trends. By incorporating AI into their underwriting practices, MGAs can differentiate themselves by offering more accurate pricing and faster turnaround times compared to traditional insurers.
2. Optimizing Claims Processing
Improving customer experience. LLMs could handle routine claims autonomously, flag potential fraud, and assist human claims specialists in resolving complex cases. Faster and more transparent claims processing will enhance client satisfaction and retention.
3. Investing in Data Infrastructure and Compliance
Effective training and use of LLMs relies heavily on high-quality, analytics-ready data – something any graduate student, researcher, and/or intern perpetually has Halloween independent nightmares about. MGAs must establish robust data management and governance frameworks to ensure that data is clean, compliant, and securely accessible. This is crucial given the increasing cybersecurity and regulatory scrutiny around AI use in insurance.
4. Building Strategic Partnership
Collaborate with insurtech (or pure AI/LLM development) companies. This will help accelerate build and adoption to provide access to this cutting-edge technology that allows for a competitive edge.
5. Start Redefining Human Roles and Training
As integrated AIs start to handle routine tasks, MGAs should reskill and upskill their workforce to focus on roles that require complex decision-making, customer interaction, and strategic thinking. This shift will be key to maintaining employee engagement and ensuring that human talent is aligned with evolving business needs.
Conclusion
AI/LLMs have the potential to revolutionize the commercial insurance industry by enhancing risk assessment, automating claims processing, and improving operational efficiency. MGAs must proactively adapt by investing in AI-driven capabilities, building robust data infrastructure, and fostering partnerships that leverage the power of these technologies. By doing so, MGAs can potentially outpace industry change, while positioning themselves as leaders within an increasingly AI-driven landscape.
Note: But wait, this is all transformer architecture, some might say!
What about deep-learning recommendation models (DLRM)?
Or academia-based models like recurrent neural networks (sequential data, e.g. weather forecasting, speech processing, natural language processing), variational auto-encoding (complex data patterning, e.g. spotting outliers, crunching high-dimensional data, image reconstruction), graph neural networks (relational data, e.g. predicting user relations, molecule-to-drug interactions) and such?
What about the mythical post-transformer architectures like state space, neuro-symbolic, and other models only three people within the field would know about?
¯\_(ツ)_/¯
Or maybe that’s just the AI speaking.
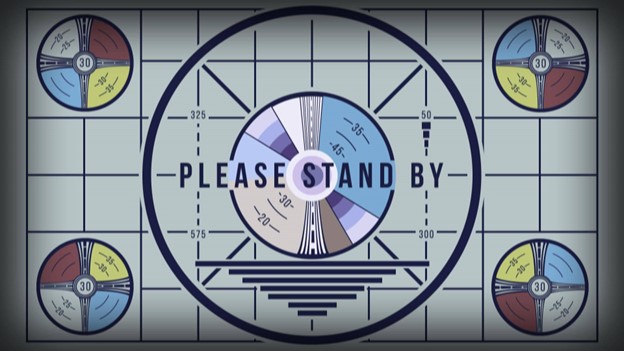
Sources:
- KPMG: The impact of artificial intelligence on the insurance industry. Accessed September 26, 2024.
- KPMG: 2024 Insurance Industry Forecast: Embracing M&A, AI, Talent, and Climate Change Mitigation. Accessed September 26, 2024.
- The Impact of AI on the Insurance Industry. Insights, Sidley Austin, LLP. Accessed September 26, 2024.
- Eloundou T, Manning S, Mishkin P, Rock D. GPTs are GPTs: An Early Look at the Labor Market Impact Potential of Large Language Models (Working Paper). arXiv:2303.10130[econ.GN], August 22, 2023. Accessed September 26, 2024.
- Accenture. Insurers: Start Boosting your “AIQ”. Accessed September 30, 2024.